Speakers
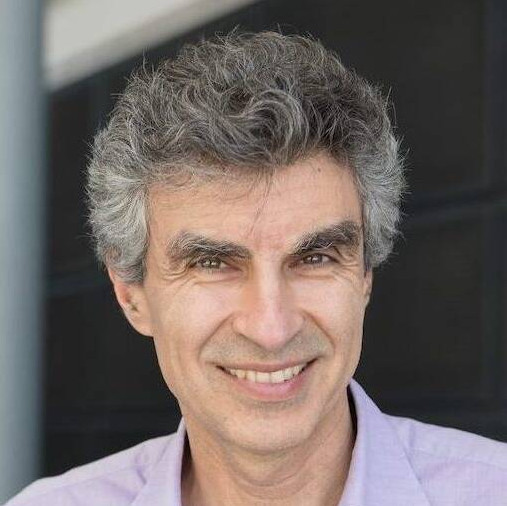
Yoshua Bengio
MILA
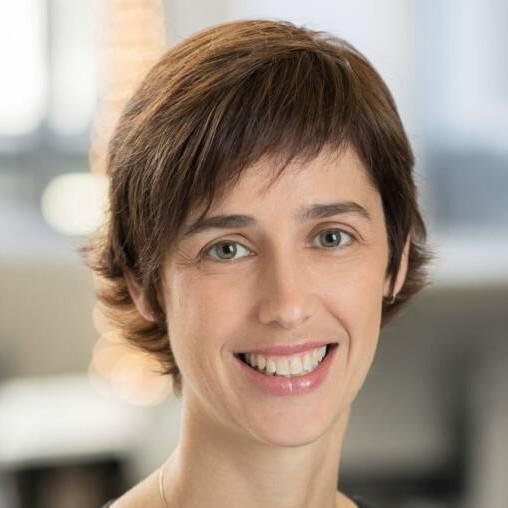
Joelle Pineau
FAIR, MILA, McGill
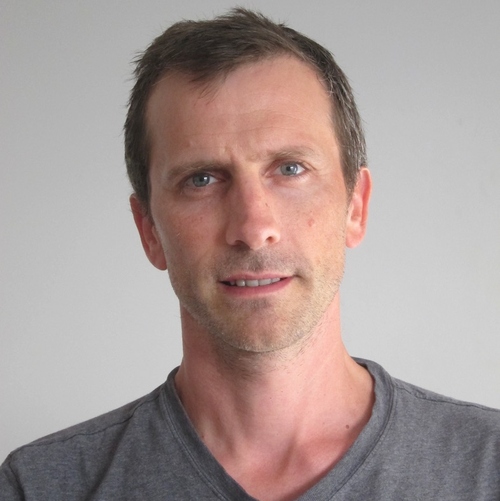
Francis Bach
INRIA
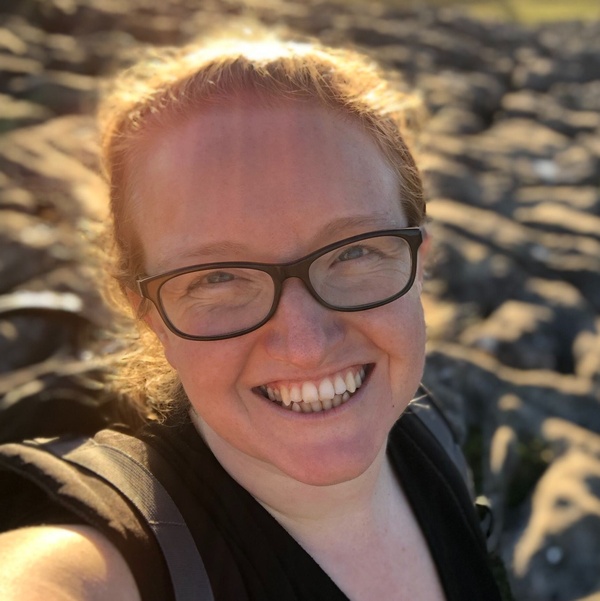
Kirstie Whitaker
Alan Turing Institute, Cambridge University
All the talks and proceedings are publicly available (see below).
MILA
FAIR, MILA, McGill
INRIA
Alan Turing Institute, Cambridge University
Benchmarks on popular datasets have played a key role in the considerable measurable progress that machine learning has made in the last few years. But reviewers can be tempted to prioritize incremental improvements in benchmarks to the detriment of other scientific criteria, destroying many good ideas in their infancy. Authors can also feel obligated to make orthogonal improvements in order to “beat the state-of-the-art”, making the main contribution hard to assess.
Pre-registration changes the incentives by reviewing and accepting a paper before experiments are conducted. The emphasis of peer-review will be on whether the experiment plan can adequately prove or disprove one (or more) hypotheses. Some results will be negative, and this is welcomed. This way, good ideas that do not work will get published, instead of filed away and wastefully replicated many times by different groups. Finally, the clear separation between hypothesizing and confirmation (absent in the current review model) will raise the statistical significance of the results.
Call for Papers: We are inviting submissions on the broad range of topics covered at NeurIPS! The paper template is structured like a mini-tutorial on the pre-registration process to get you started quickly. Pre-registered papers will be published at the workshop. Authors will then have the opportunity to submit the results paper to the Proceedings of Machine Learning Research (PMLR), a sister publication to the Journal of Machine Learning Research (JMLR). The review process for this second stage will aim to ensure that the authors have performed a good-faith attempt to complete the experiments described in their proposal paper.
1) Proposal phase: | Selection of pre-registered papers for the NeurIPS Workshop |
9th Oct 2020 | Paper submission (authors) |
17th Oct 2020 | Reviews due (reviewers) |
19th Oct 2020 | Reviews available to authors |
22nd Oct 2020 | Rebuttal due (authors) |
27th Oct 2020 | Final reviews due (reviewers) |
31st Oct 2020 | Notification of decisions |
27th Nov 2020 | Camera ready submission (authors) |
11th Dec 2020 | NeurIPS 2020 workshop day |
2) Results phase: | Selection of results papers for PMLR |
7th May 2021 | Paper submission (authors) |
7th June 2021 | Reviews due (reviewers) |
14th June 2021 | Notification of decisions |
28th June 2021 | Camera ready submission (authors) |
8th July 2021 | PMLR volume published: http://proceedings.mlr.press/v148/ |
Playlist of all 1-minute preview videos
ID | Authors | Title | Proposal | Video | Poster | Results |
5 | Kexue Fu, Xiaoyuan Luo, Manning Wang | Point Cloud Overlapping Region Co-Segmentation Network | Proposal | Video | Poster | Results |
7 | Udo Schlegel, Daniela Oelke, Daniel Keim, Mennatallah El-Assady | An Empirical Study of Explainable AI Techniques on Deep Learning Models For Time Series Tasks | Proposal | Video | Poster | |
17 | Akshay L Chandra, Sai Vikas Desai, Chaitanya Devaguptapu, Vineeth N Balasubramanian | On Initial Pools for Deep Active Learning | Proposal | Video | Poster | Results |
19 | Liu Yuezhang, Bo Li, Qifeng Chen | Evaluating Adversarial Robustness in Simulated Cerebellum | Proposal | Video | Poster | Results |
21 | XueHao Gao, Yang Yang, Shaoyi Du | Contrastive Self-Supervised Learning for Skeleton Action Recognition | Proposal | Video Oral | Poster | Results |
26 | Ayush Jaiswal, Yue Wu, Pradeep Natarajan, Prem Natarajan | Keypoints-aware Object Detection | Proposal | Video | Poster | Results |
27 | Cade Gordon, Natalie Parde | Latent Neural Differential Equations for Video Generation | Proposal | Video | Poster | Results |
28 | Robert Vandermeulen, Rene Saitenmacher, Alexander Ritchie | A Proposal for Supervised Density Estimation | Proposal | Video | Poster | |
31 | Eimear O' Sullivan, Stefanos Zafeiriou | PCA Retargeting: Encoding Linear Shape Models as Convolutional Mesh Autoencoders | Proposal | Video Oral | Poster | Results |
33 | Rasmus Palm, Elias Najarro, Sebastian Risi | Testing the Genomic Bottleneck Hypothesis in Hebbian Meta-Learning | Proposal | Video Oral | Poster | Results |
36 | Rodrigo Alves, Antoine Ledent, Renato Assunção, Marius Kloft | An Empirical Study of the Discreteness Prior in Low-Rank Matrix Completion | Proposal | Video | Poster | Results |
38 | Elena Burceanu | SFTrack++: A Fast Learnable Spectral Segmentation Approach for Space-Time Consistent Tracking | Proposal | Video | Poster | Results |
39 | Rishika Bhagwatkar, Khurshed Fitter, Saketh Bachu, Akshay Kulkarni, Shital Chiddarwar | Paying Attention to Video Generation | Proposal | Video | Poster | Results |
40 | Chen Li, Xutan Peng, Hao Peng, Jianxin Li, Lihong Wang, Philip Yu, | TextSGCN: Document-Level Graph Topology Refinement for Text Classification | Proposal | Video | Poster | |
41 | Chase Dowling, Ted Fujimoto, Nathan Hodas | Policy Convergence Under the Influence of Antagonistic Agents in Markov Games | Proposal | Video Oral | Poster | |
42 | Arnout Devos ,Yatin Dandi | Model-Agnostic Learning to Meta-Learn | Proposal | Video | Poster | Results |
44 | Carianne Martinez, Adam Brink, David Najera-Flores, D. Dane Quinn, Eleni Chatzi, Stephanie Forrest, | Confronting Domain Shift in Trained Neural Networks | Proposal | Video Oral | Poster | Results |
45 | Joao Monteiro, Xavier Gibert, Jianqiao Feng, Vincent Dumoulin, Dar-Shyang Lee | Domain Conditional Predictors for Domain Adaptation | Proposal | Video | Poster | Results |
47 | Tanner Bohn, Xinyu Yun, Charles Ling | Towards a Unified Lifelong Learning Framework | Proposal | Video | Poster | Results |
48 | Hamid Eghbal-zadeh, Florian Henkel, Gerhard Widmer | Context-Adaptive Reinforcement Learning using Unsupervised Learning of Context Variables | Proposal | Video | Poster | Results |
50 | Aneesh Dahiya, Adrian Spurr, Otmar Hilliges | Exploring self-supervised learning techniques for hand pose estimation | Proposal | Video | Poster | Results |
55 | Sebastian Stabinger, David Peer, Antonio Rodriguez-Sanchez | Training of Feedforward Networks Fails on a Simple Parity-Task | Proposal Supmat | Video | Poster | |
56 | Pablo Barros, Ana Tanevska, Ozge Nilay Yalcin, Alessandra Sciutti | Incorporating Rivalry in Reinforcement Learning for a Competitive Game | Proposal | Video | Poster | |
57 | Steffen Schneider, Shubham Krishna, Luisa Eck, Wieland Brendel, Mackenzie Mathis, Matthias Bethge | Generalized Invariant Risk Minimization: relating adaptation and invariant representation learning | Proposal Supmat | Video | Poster | |
58 | Alex Lewandowski | Generalization Across Space and Time in Reinforcement Learning | Proposal | Video | Poster | |
59 | Prabhu Pradhan, Ruchit Rawal, Gopi Kishan | Rendezvous between Robustness and Dataset Bias: An empirical study | Proposal | Video | Poster | |
60 | Miles Cranmer, Peter Melchior, Brian Nord | Unsupervised Resource Allocation with Graph Neural Networks | Proposal | Video Oral | Poster | Results |
62 | Swaroop Mishra, Anjana Arunkumar, Bhavdeep Sachdeva | Is High Quality Data All You Need? | Proposal | Video | Poster | |
67 | Yi-Fan Li, Yang Gao, Yu Lin, Zhuoyi Wang, Latifur Khan | Time Series Forecasting Using a Unified Spatial-Temporal Graph Convolutional Network | Proposal Supmat | Video | Poster | |
69 | Norman Tasfi, Eder Santana, Miriam Capretz | Policy Agnostic Successor Features | Proposal | Video | Poster | |
71 | Owen Lockwood, Mei Si | Playing Atari with Hybrid Quantum-Classical Reinforcement Learning | Proposal | Video | Poster | Results |
76 | Ajinkya Mulay, Ayush Manish Agrawal, Tushar Semwal | FedPerf: A Practitioners' Guide to Performance of Federated Learning Algorithms | Proposal | Video Oral | Poster | Results |
77 | Harshvardhan Sikka, Atharva Tendle, Amr Kayid | Multimodal Modular Meta-Learning | Proposal | Video | Poster | |
79 | Philipp Benz, Chaoning Zhang, Adil Karjauv, In So Kweon | Robustness May Be at Odds with Fairness: An Empirical Study on Class-wise Accuracy | Proposal | Video | Poster | Results |
81 | Ruizhe Li, Xutan Peng, Chenghua Lin, Frank Guerin, Wenge Rong | On the low-density latent regions of VAE-based language models | Proposal | Video Oral | Poster | Results |
82 | Pratyush Kumar, Aishwarya Praveen Das, Debayan Gupta | Differential Euler: Designing a Neural Network approximator to solve the Chaotic Three Body Problem | Proposal | Video | Poster | |
83 | Jiaqi Fan, Junxin Huang, Xiaochuan Yu, Chao He | Data Subset Selection for Object Detection | Proposal | Video | Poster | |
85 | Meenakshi Sarkar, Debasish Ghose | Decomposing camera and object motion for an improved Video Sequence Prediction | Proposal | Video | Poster | Results |
Many thanks to all the reviewers for their help!